AI Edge Cases: Google, Cars & Bots
AI Gaffes: Google,Uber & Chatbots
A few days ago, I announced that Chris Kieff and I are writing a new book and I warned that our working title may keep changing. It already has. We’ve updated our book title already to AI Edge Cases—How Little Stuff Can Ruin Revenue & Reputation.
What do you think?
We also asked readers for comments and questions and got a few really good ones. Paul Walhus wanted us to define the term edge case term more clearly and Laurel Papworth requested specific examples.
Responding first to Paul, an edge case is a term software professionals use to describe unusual events that break existing processes and procedures causing significant losses of time, money, reputation, and customers. Our book focuses just on cases that cause AI systems to fail.
Addressing Laurel’s question requires a more editorial space. Case studies will make up a significant chunk of this book. We’re just getting started and we’ve only researched about a dozen cases so far, but we expect a significant portion of this book will spotlight case studies. This book is design to educate and alert business decision makers of the magnitude of the AI edge case problem and we have no easy solutions to that problem. However: we can help executives to be better informed and case studies play a big part in that.
Here are three examples that we will use, and I hope it gives readers ideas of what we are looking for and looking at. The cases, I have picked scare the Hell out of me, but I have no desire to be a sensationalist: I do want to point out the severity of the AI edge case problem.
1. Google Gaffes
Google is a pioneer and leader in Machine Learning and Pattern Recognition and other AI categories. Most of us use their software daily taking it for granted as we benefit from Search, Photos and Bard, ChatGPT’s most formidable competitor.
According to Bard, Google's search engine processes over 8.5 billion searches per day, which comes out to over 2 trillion searches per. Most of us just take these services for granted because they are reliable 97.5 percent of the time.
Google is a leader in Machine Learning and Pattern Recognition, as well as other AI categories. Most of us use their software daily taking it for granted as we use the benefits of Search, Photos and Bard, ChatGPT’s most formidable competitor. Almost always, Google software gives users what they are searching for and that makes us automatically trust its products.
But that other 2.5 percent of the time we get goofy answers that that amuse or outrage us are the AI edge cases that we are addressing.
For example:
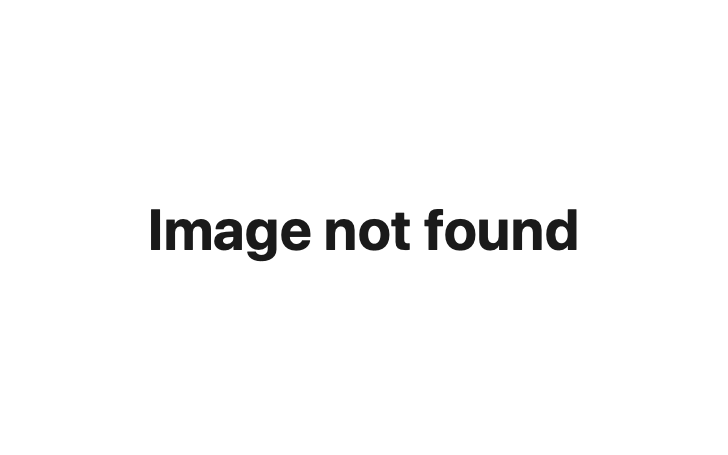
But sometimes it gets worse--a lot worse--serving as a dent in a brand’s image that remains for years. You may recall a 2015 incident when a flawed facial recognition algorithm misclassified photos of black people as gorillas. You probably remember the incident from eight years ago and you will continue to do so for years to come.
It was a well-publicized gaffe that still haunts the company eight years later and has been amplified by other AI-generated mishaps such as an image of a traditional Thanksgiving dinner that featured a large potato where a turkey should have appeared. It has also recorded stumbles for misidentifying the World Trade Center and the face of Abraham Lincoln.
Here is a company that has spent hundreds of billions of dollars, perhaps more than any other company on AI. It has correctly responded to trillions of requests on its AI platforms and yet it is treated by many on social traditional media as a virtual punching bag.
The company has taken many steps to fix what was broken in its AI to prevent recurrences, and yet it is still paying a price for the gorilla incident that was bolstered by the other three instances.
Here’s a partial list of their actions:
● Almost immediately after the Gorilla incident, Sundar Pichai, Google’s CEO, stopped what he was doing to call a news conference to apologize and promise such mistakes would not recur.
● Google’s communications teams have invested countless hours and many dollars on brand repair.
● The tech team started working on algorithmic adjustments, blocked its Photos app from showing photos of any primates except humans, until it could correctly identify each species.
● The company has invested heavily in getting the corporate culture to prioritize ethics and has vigorously endeavored to stop or minimize machine bias and it has improved Testing Protocols.
● It has invested countless millions in developing processes for preventing AI edge cases but understand that the laws of probability say they will happen again, so they have developed action plans on what to do when more stuff hits the fan.
● Google has historically been perceived as a top career choice for some of the world’s best and brightest recent graduates. Some may not want to join a company that portrays Black as gorillas.
We cannot estimate the full cost of Google’s gaffes, nor will we hazard guesses on how long the company will continue pay for that one embarrassing edge case in 2015, but we are certain that it is a much higher number than that 2.5 percent we cited.
Edge cases are often dismissed because the odds make them seem like a longshot but covering that bet can be very significant.
2. Autonomous Edges.
In 2018, an autonomously driven Volvo SUV was being tested by Uber in Tempe, Az, when it struck and killed Elaine Herzberg as she walked her bicycle across a dark street outside the designated crosswalk. But the AI’s training hadn’t anticipated someone walking a bicycle in the dark and outside of a crosswalk so the system failed to respond.
The Uber’s failsafe driver in the Volvo to take control in precisely such a situation. He later testified that he had seen Herzberg but was sure the Volvo would handle it until 1.3 seconds before the she was struck.
The chances of an autonomous car striking a pedestrian walking a bike outside of a crosswalk are extremely low, making this an edge case that has damaged trust in autonomous cars and may slow user adoption.
Perhaps it should: Chesley “Sully” Sullenberger II, the pilot who in 2009 lost engine power on take off from LaGuardia Airport then saved 153 lives by refusing a computer directive to land at a New Jersey airport, choosing instead to land it on the Hudson River creating the famous Miracle on the Hudson, which was a rare story where an edge case had a happy outcome.
More recently, Sully spoke strongly against autonomous cars, warning that unlike the decisions he had to make, there were too many “unknown unknowns” in driving cars on crowded highways. The term is derived from risk management, and refers to the process of identifying, assessing, and controlling financial, legal, strategic and security risks and other factors that could lead to management errors, accidents. natural disasters and software glitches, as ChatGPT uses it.
I very much doubt that even the best risk manager could calculate the odds against Elaine Herzberg being struck and killed by a self-driving Volvo, but that’s a key point to our book: edge cases all enterprises but AI Edge Changes are the most damaging and expensive.
3. Chatbot Angst
Chatbots have been around since the 1990s, and it is likely that you have already used them for help in support, purchasing, health, and education. Perhaps you have had a conversation with Google Assistant, Amazon Alexa, or Siri, all of whom use natural language recognition, a form of AI that lets people talk with machines like them.
Companies love chatbots because they reduce customer support costs and increase efficiency. Customers are often satisfied with the help they get while some of us still yearn for the help of humans.
Usually, they work just fine, but about 2-3 percent of the time their answers are just lame. Sometimes the mistakes are caused by user errors or people who speak with accents that the software was not taught to understand or it may be an algorithm with hiccups. When we communicate via keyboards, we sometimes make typos or requests that are too complex for the AI to understand, so the machine replies with answers that seem to be inane or just dead wrong.
When chatbots go lame on us, it can confuse or infuriate us. We’re paying customers and we are trying using products as we believe we are supposed to do and we just need something fixed so we can resume our regularly scheduled lives and we ask the bot a question and get a response that has nothing to do with anything or suggest we buy a new product from the company that is currently frustrating us.
Me? I sometimes find myself screaming at inanimate digital objects or type in all caps. I picture the decision makers who chose profitable automated efficiencies over serving customers like me and I m unlikely to ever be a trusting customer again.
My only revenge will be my certainty that sooner or later, a competitor will develop AI that is more responsive than that company has been.
These are just three of several examples we will discuss in the book. We are looking for many more of them for our book. Our thanks again to Laurel and Paul for asking these two questions. They will be acknowledged in Edge Cases when we publish. If you point us in the direction of other good stories, companies and thoughts related to AI edge cases, please leave a comment or email me. Also, we are looking for sponsors for this project please contact Shel for further information.
++++++